Introduction
Cohere introduced its next-generation foundation model, Rerank 3 for efficient Enterprise Search and Retrieval Augmented Generation(RAG). The Rerank model is compatible with any kind of database or search index and can also be integrated into any legal application with native search capabilities. You won’t imagine, that a single line of code can boost the search performance or reduce the charge of running an RAG application with negligible impact on latency.
Let’s explore how this foundation model is set to advance enterprise search and RAG systems, with enhanced accuracy and efficiency.
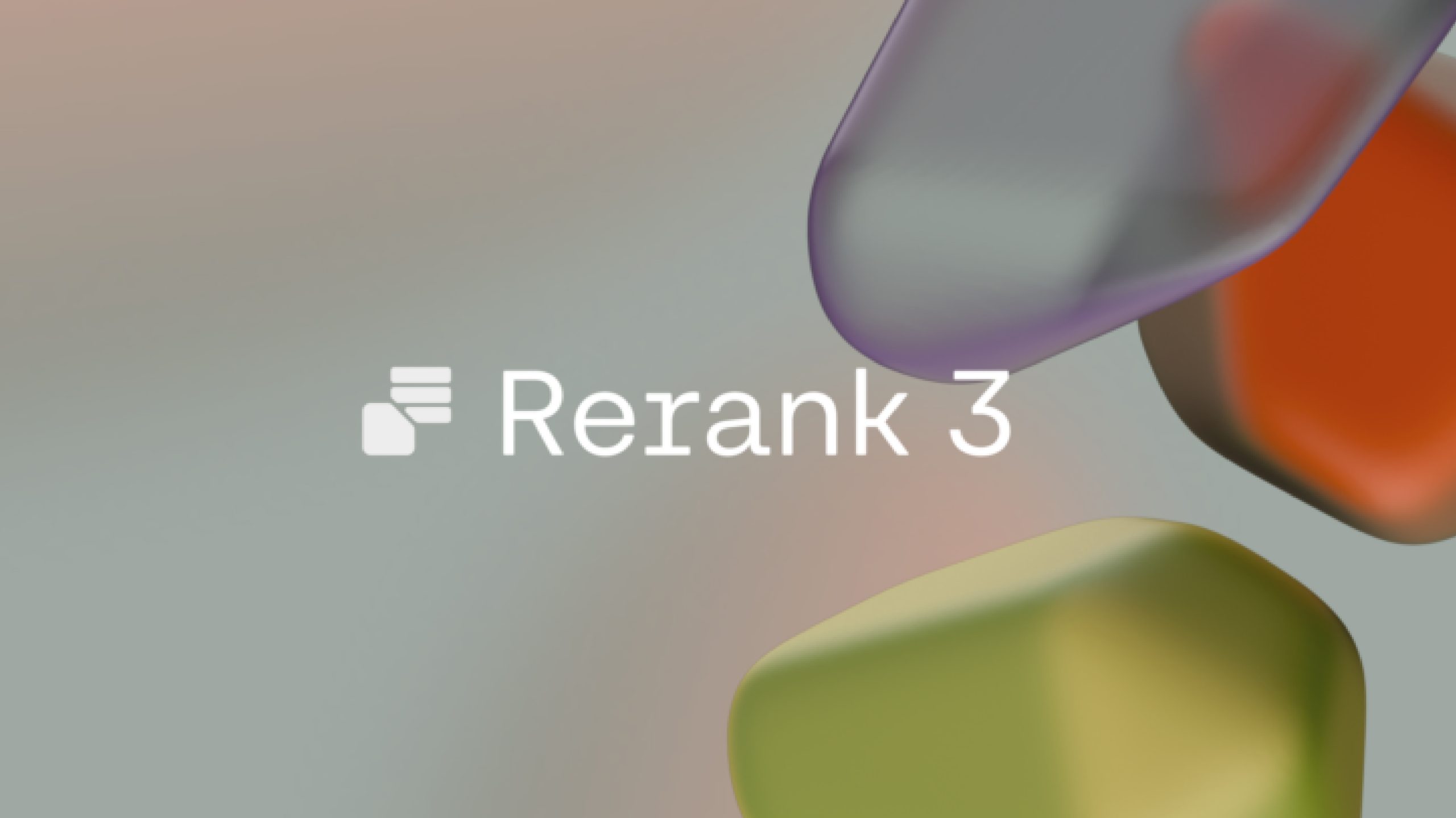
Capabilities of Rerank
Rerank offers the best capabilities for enterprise search which include the following:
- 4K context length which significantly enhances the search quality for longer-form documents.
- It can search over multi-aspect and semi-structured data like tables, code, JSON documents, invoices, and emails.
- It can cover more than 100 languages.
- Enhanced latency and decreased total cost of ownership(TCO)
Generative AI models with long contexts have the potential to execute an RAG. In order to enhance the accuracy score, latency, and cost the RAG solution must require a combination of generation AI models and of course Rerank model. The high precision semantic reranking of rerank3 makes sure that only the relevant information is fed to the generation model which increases response accuracy and keeps the latency and cost very low, in particular when retrieving the information from millions of documents.
Enhanced Enterprise Search
Enterprise data is often very complex and the current systems that are placed in the organization encounter difficulties searching through multi-aspect and semi-structured data sources. Majorly, in the organization the most useful data are not in the simple document format such as JSON is very common across enterprise applications. Rerank 3 is easily able to rank complex, multi-aspect such as emails based on all od their relevant metadata fields, including their recency.
Rerank 3 significantly improves how well it retrieves code. This can boost engineer productivity by helping them find the right code snippets faster, whether within their company’s codebase or across vast documentation repositories.
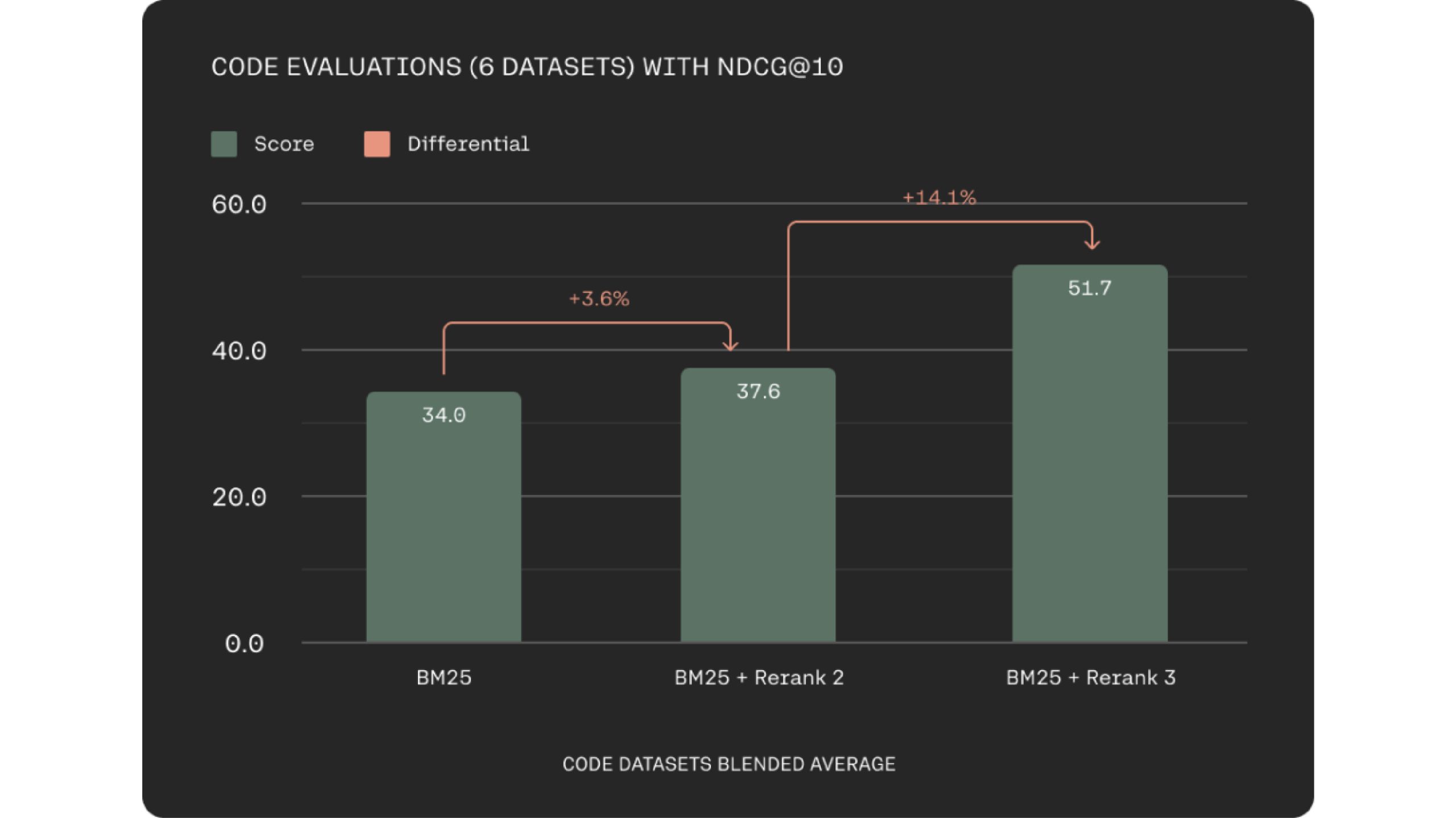
Tech giants also deal with multilingual data sources and previously multilingual retrieval has been the biggest challenge with keyword-based methods. The Rerank 3 models offer a strong multilingual performance with over 100+ languages simplifying the retrieval process for non-English speaking customers.
A key challenge in semantic search and RAG systems is data chunking optimization. Rerank 3 addresses this with a 4k context window, enabling direct processing of larger documents. This leads to improved context consideration during relevance scoring.
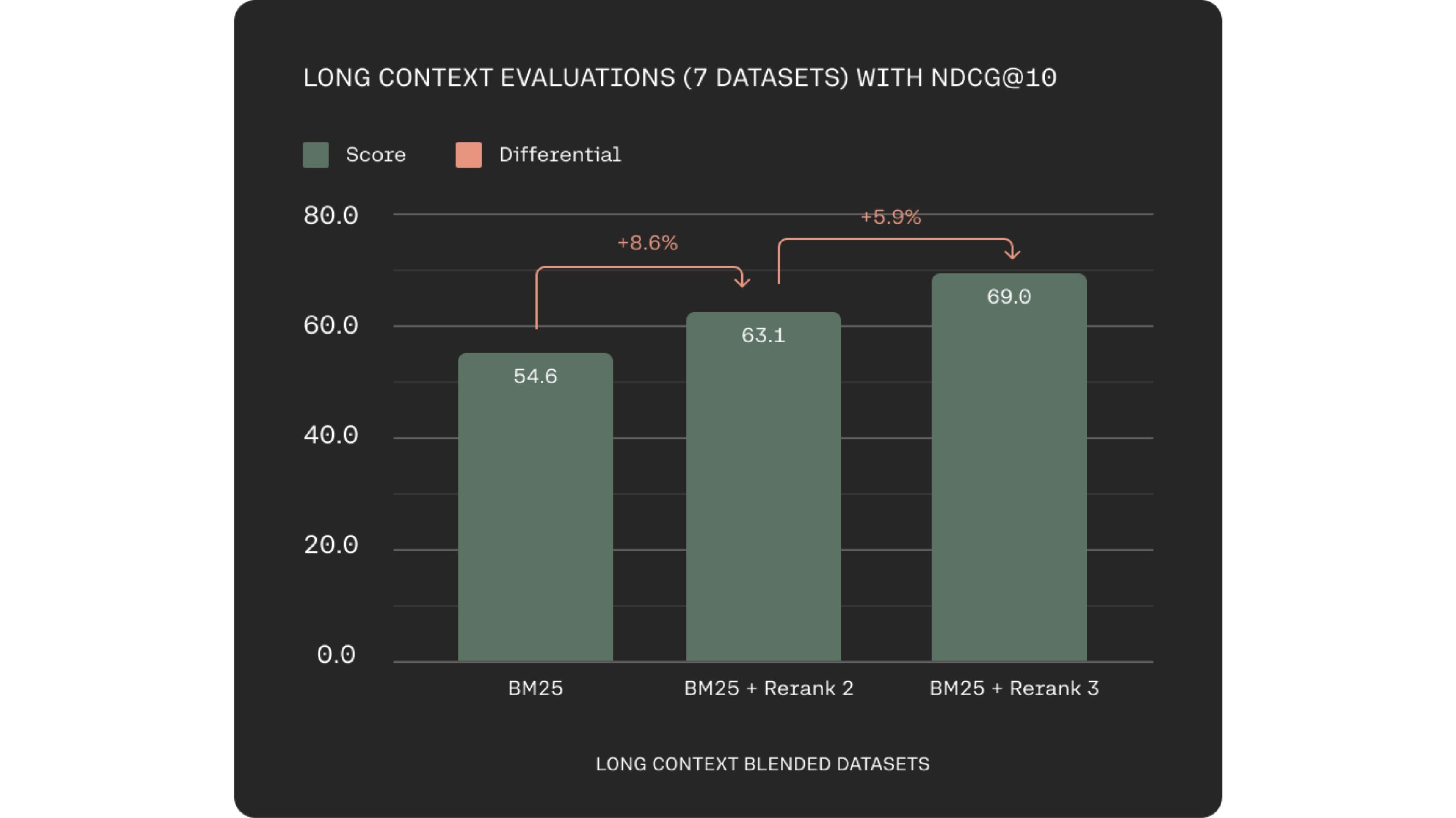
Rerank 3 is supported in Elastic’s Inference API also. Elastic search has a widely adopted search technology and the keyword and vector search capabilities in the Elasticsearch platform are built to handle larger and more complex enterprise data efficiently.
“We are excited to be partnered with Cohere to help businesses to unlock the potential of their data” said Matt Riley, GVP and GM of Elasticsearch. Cohere’s advanced retrieval models which are Embed 3 and Rerank 3 offer an excellent performance on complex and large enterprise data. They are your problem solver, these are becoming essential components in any enterprise search system.
Improved Latency with Longer Context
In many business domains such as e-commerce or customer service, low latency is crucial to delivering a quality experience. They kept this in mind while building Rerank 3, which shows up to 2x lower latency compared to Rerank 2 for shorter document lengths and up to 3x improvements at long context lengths.
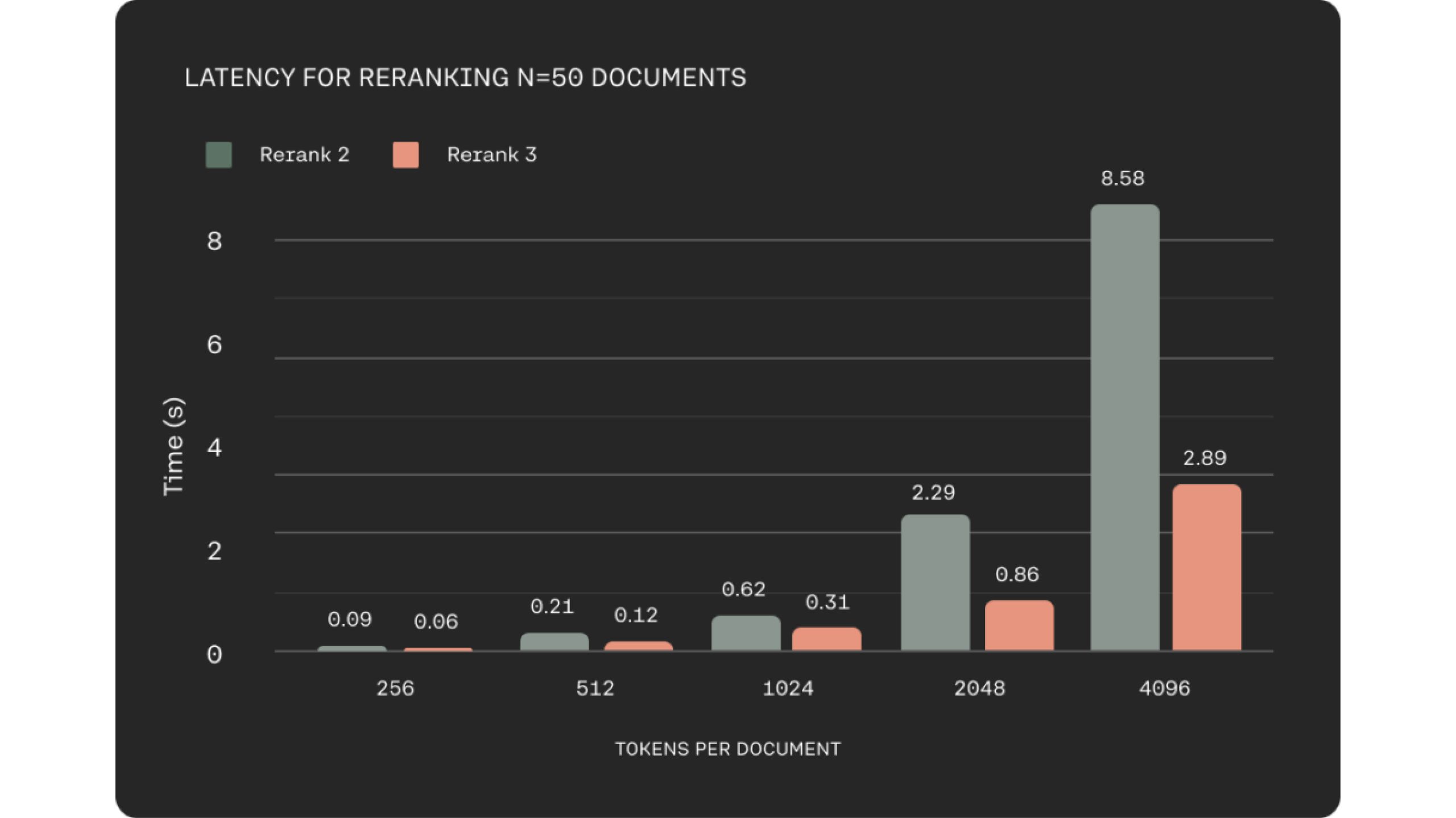
Better Performace and Efficient RAG
In Retrieval-Augmented Generation (RAG) systems, the document retrieval stage is critical for overall performance. Rerank 3 addresses two essential factors for exceptional RAG performance: response quality and latency. The model excels at pinpointing the most relevant documents to a user’s query through its semantic reranking capabilities.
This targeted retrieval process directly improves the accuracy of the RAG system’s responses. By enabling efficient retrieval of pertinent information from large datasets, Rerank 3 empowers large enterprises to unlock the value of their proprietary data. This facilitates various business functions, including customer support, legal, HR, and finance, by providing them with the most relevant information to address user queries.
Integrating Rerank 3 with the cost-effective Command R family for RAG systems offers a significant reduction in Total Cost of Ownership (TCO) for users. This is achieved through two key factors. Firstly, Rerank 3 facilitates highly relevant document selection, requiring the LLM to process fewer documents for grounded response generation. This maintains response accuracy while minimizing latency. Secondly, the combined efficiency of Rerank 3 and Command R models leads to cost reductions of 80-93% compared to alternative generative LLMs in the market. In fact, when considering the cost savings from both Rerank 3 and Command R, total cost reductions can surpass 98%.
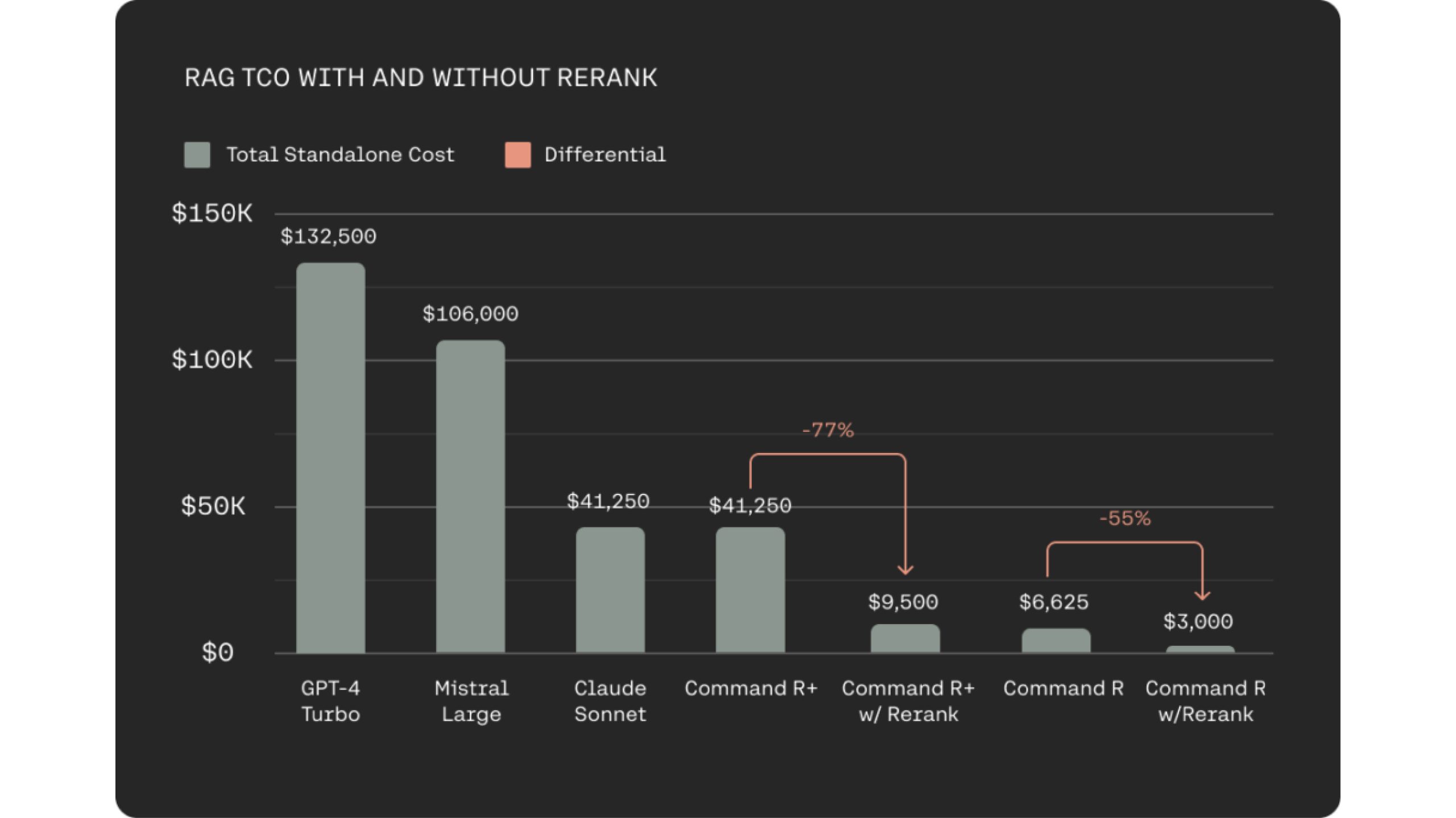
One increasingly common and well-known approach for RAG systems is using LLMs as rerankers for the document retrieval process. Rerank 3 outperforms industry-leading LLMs like Claude -3 Sonte, GPT Turbo on ranking accuracy while being 90-98% less expensive.
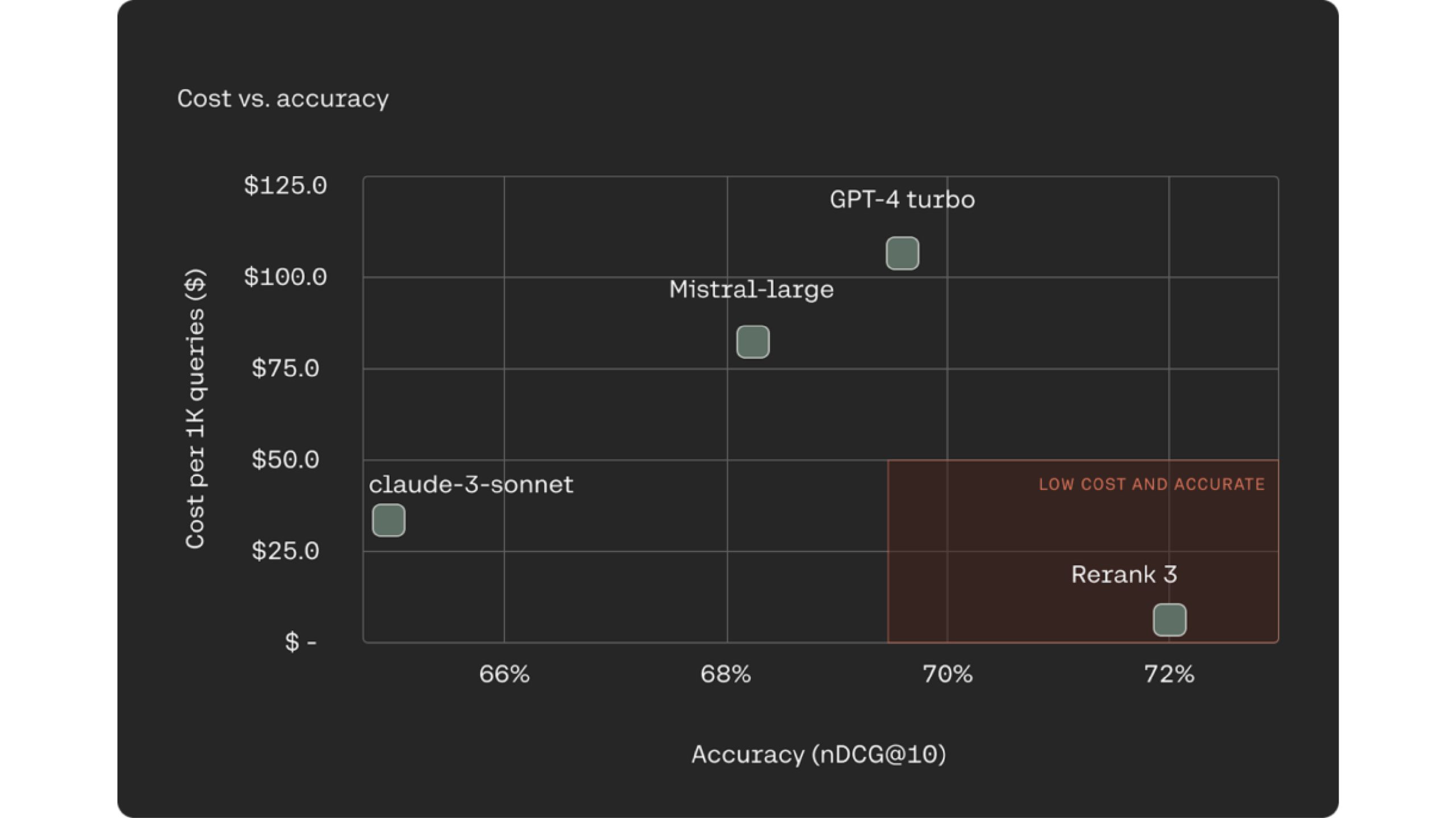
Rerank 3 boost the accuracy and the quality of the LLM response. It also helps in reducing end-to-end TCO. Rerank achieves this by weeding our less relevant documents, and only sorting through the small subset of relevant ones to draw answers.
Conclusion
Rerank 3 is a revolutionary tool for enterprise search and RAG systems. It enables high accuracy in handling complex data structures and multiple languages. Rerank 3 minimizes data chunking, reducing latency and total cost of ownership. This results in faster search results and cost-effective RAG implementations. It integrates with Elasticsearch for improved decision-making and customer experiences.
You can explore many more such AI tools and their applications here.